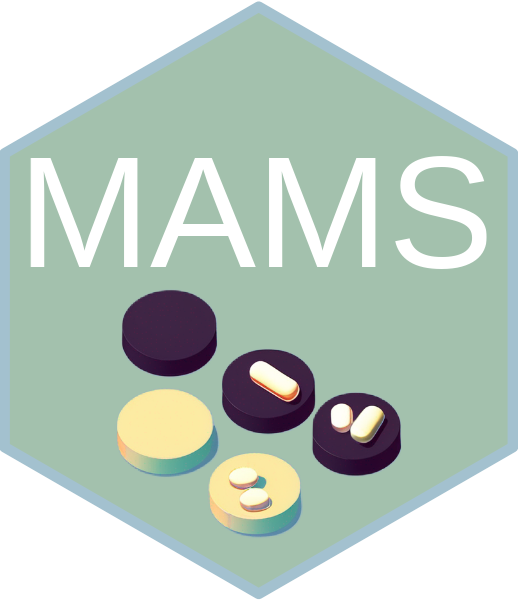
Desing operating charachteristics
evaluating_properties.Rmd
Design operating characteristics to evaluate the properties of a
particular design via simulation the function mams.sim
can
be used. It allows for flexible numbers of subjects per arm and stage in
the form of a J
× (K
+ 1) matrix
nMat
. In addition to the upper and lower boundaries
(u
and l
), a vector of true success
probabilities (pv
) is required (or alternatively a vector
of true effect sizes (deltav
) and a standard deviation
(sd
)). The parameter ptest
allows to specify
rejection of which hypotheses should be counted in the power
calculation. We evaluate the properties of the two-stage design
m2
under the global null hypothesis (i.e., a true effect
size of \(p = 0.5\) or \(δ = 0\) for all treatments) with 100,000
simulation runs.
The probability of rejecting at least one hypothesis is 0.050, and
since we simulated under the global \(H_0\), this corresponds to a
FWER of 5% as desired. The power to reject the first
hypothesis when it has the largest estimated effect is 0.016, and the
power to reject either \(H_1\) or \(H_2\) or both of them (as specified by
ptest = 1:2
) is 0.034. The expected number of patients
required for the trial under the global \(H_0\) is 244.7 in contrast to the maximum
required of 380. The function mams.sim
is also useful to
simulate and compare expected sample sizes of different designs. We
illustrate this for the designs poc
, obf
and
tri
(whose boundaries are shown in Multi-stage design) under the LFC of
the alternative, i.e., one treatment’s effect size equals.
Design | Minimum | Maximum | Expected (LFC) | Expected (H0) |
---|---|---|---|---|
Pocock | 132 | 396 | 232.2 | 385.6 |
O'Brien-Fleming | 112 | 336 | 259.1 | 322.0 |
Triangular | 136 | 408 | 217.8 | 222.1 |
\(p = 0.65\) whereas the effect sizes for all other treatments are equal to \(p_0 = 0.55\), using 100,000 simulation runs.
pocsim <- mams.sim(nsim = 1e5, nMat = t(poc$n * poc$rMat), u = poc$u, l = poc$l,
pv = c(0.65, rep(0.55, 2)), ptest = 1)
obfsim <- mams.sim(nsim = 1e5, nMat = t(obf$n * obf$rMat), u = obf$u, l = obf$l,
pv = c(0.65, rep(0.55, 2)), ptest = 1)
trisim <- mams.sim(nsim = 1e5, nMat = t(tri$n * tri$rMat), u = tri$u, l = tri$l,
pv = c(0.65, rep(0.55, 2)), ptest = 1)
Similarly, we can obtain the design properties under the global null
hypothesis by setting pv = rep(0.5, 3)
. Table summarizes
minimum, maximum, and expected sample sizes of the three designs. We see
that under both the LFC and the global \(H_0\) the triangular design is expected to
require the lowest number of patients. On the other hand,
O’Brien-Fleming has the lowest minimum and maximum but the highest
expected sample size under the LFC of all three designs. Under the
global \(H_0\) both the Pocock and
O’Brien-Fleming designs have expected sample sizes that are very close
to their respective maxima.